Better account prioritization
Your plan is set, and your territories are divvied up. How do you make sure your sales team is working as efficiently as possible?
This free guide details everything you need to prioritize accounts for outreach. Ensure reps are always working the highest potential accounts in their books.
Our new account prioritization guide includes discussion on fit and timing, intent signals, ICP definition, and account scoring.
Keep scrolling to read through the guide.
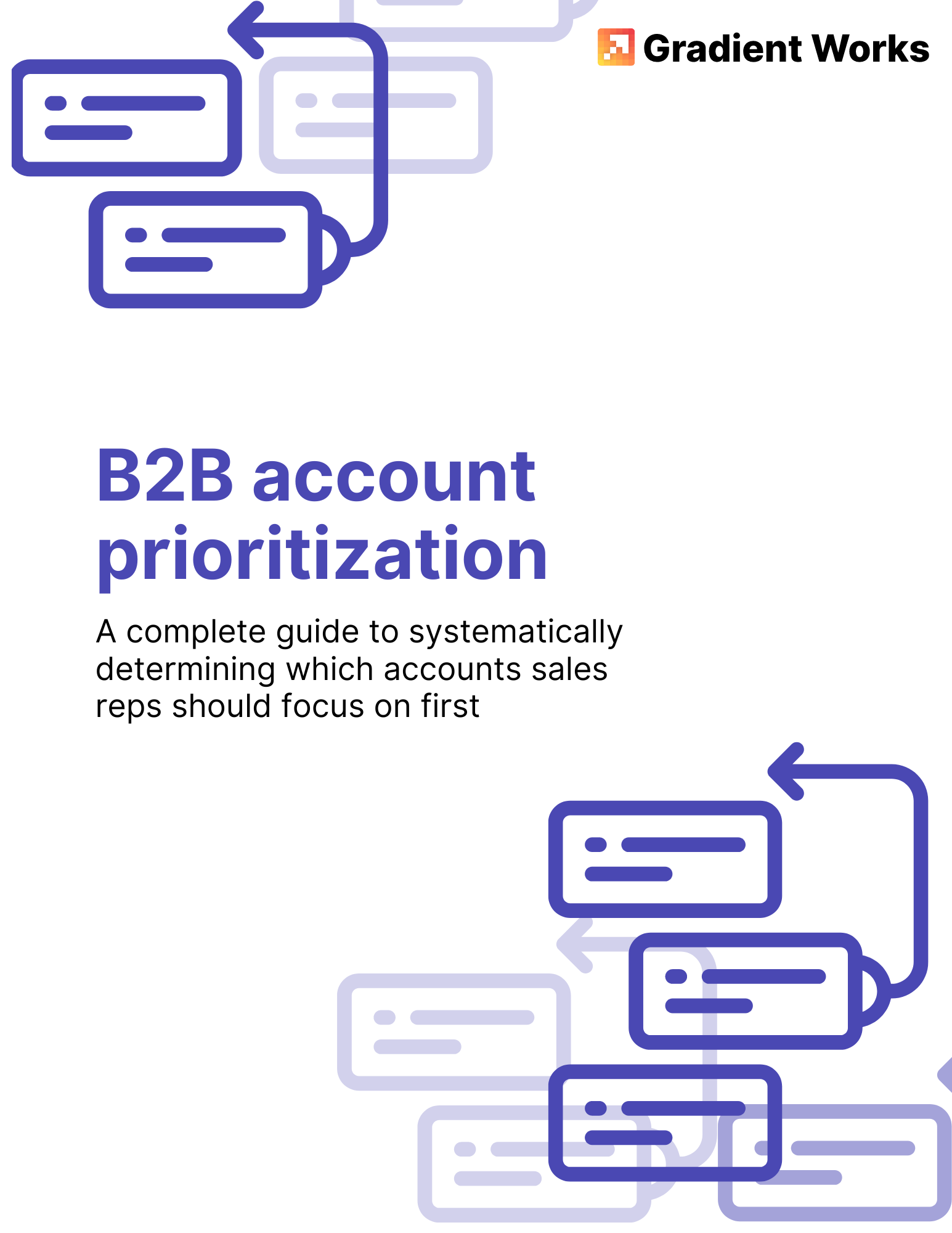
Account prioritization
Even in a good year, 69% of salespeople don’t have enough leads in their pipeline to hit quota (source). It’s worse now, as inbound has slowed down and outbound is harder. Right now, reps need more top of funnel pipeline to hit their opportunity creation goals.
In addition, up to half of sales performance can be explained by a rep’s territory potential versus their sales skills (source). Even a great rep can’t sell to a bad territory.
So, just stop giving good reps bad accounts. Okay, if only it were that easy! Deciding what comprises a seller’s book of accounts is a lot more complicated than just divvying up a list of accounts and handing them to a salesperson to work.
The guide that follows is going to show you how to make sure every sales rep on your team is calling on the best possible accounts in your market, and how you can implement these methods now, even after your 2024 territories have been set.
Once you’ve segmented your market and rolled out your new territories or updates books, you need to help reps prioritize which accounts or segments to work first. Are there certain accounts that are more likely to buy now? How do you identify those? How do sales reps know which accounts to focus on first in their new territories?
Account prioritization is systematically determining which accounts have the highest potential and should be worked first.
Fit and timing
Most companies have a definition of what makes a prospect account a good fit (“this kind of account is a fit for our solution”), as well as what elements signal the right timing for outreach (“when should we reach out for maximum impact?”). Let’s talk more about each of those and how they work together to help you prioritize the highest potential accounts.
First, please meet the fit and timing quadrant.
The fit and timing quadrant helps clarify how much attention you pay to an account, depending on its fit and timing qualifications. Every account should fall into one of four quadrants.
The Good Place. These accounts are both a great fit and they’re ready to buy. These are the opportunities you can win in the short term and ideally where your reps are spending most of their time. This is the zone to win.
Get Back to Me (GBTM) Zone. These accounts are a great fit but the timing isn’t right (yet). They may be under contract with a different vendor or have another major priority this quarter. When you talk to them, they’ll probably say something like “This sounds great, but let’s talk next quarter.” This is valuable information you can use later, and you should absolutely be keeping track of that. More on that in a bit.
False Hope Zone. These accounts are a bad fit even though they’re currently looking for a solution. In fact, you’ll probably even win some of these - mostly because they’re ready to buy something similar to what you offer. But, you’re likely to find that you can’t repeat this process, and/or they’re bad customers who will be difficult to service and likely to churn. These will often come through your inbound demand gen channel and are tricky because reps will hear only the good news about timing and ignore the bad fit signals.
The Bad Place. These accounts aren’t a fit and they’re not looking to buy. Hopefully, you’ll avoid these altogether, but it’s more likely that your team will waste time on a sales cycle with no chance of success. These frequently arise from poor prospecting. If you have a geographic territory model, reps are in danger of spending a lot of time here unless they’re excellent at discovery and qualification. With a little ops work, you can keep reps out of The Bad Place entirely. More on that as we go.
Accounts can move between quadrants, though your ability to influence that movement is limited. Most fit and timing criteria are beyond your control. All you can control is how and when you connect with those accounts.
Timing signals change predictably over time. Accounts will move back and forth between being in market and not in market as they buy solutions, renew solutions, and go through budget cycles. Given enough information, you can probably predict most of these moves, but there’s not much you can do to change an account’s readiness-to-buy timing.
Fit rarely changes. However, you may find accounts that grow into (or fall out of) your ICP, either through organizational changes or acquisitions, or periods of significant growth. You may find new accounts in your ICP if you start selling a new product or venturing into new markets. Lots more on ICP shortly.
Pipeline starts with account coverage based on both fit and timing
As a sales leader, it's your job to focus rep effort on the best fit, highest timing accounts in your SOM. Ensuring effective account coverage is a shared responsibility between marketing, ops and sales.
Account coverage requires continuous adjustment. You have to actively manage coverage all the time; it’s no longer sufficient to relegate this to a once-a-year territory planning process. You may divvy up your territories once a year (though of course, we think you should switch to a dynamic books model), but you should be continuously managing which accounts reps are working in their territories.
We're going to go into a ton of detail about account scoring, understanding ICP fit, and intent and timing signals in a moment. But before we get to that, here are a few ways to improve account coverage:
1. Integrate rep-level account coverage analysis into every 1:1. Help reps learn how to better cover the best accounts in their books or territories. Show them which accounts to focus on and which to ignore.
2. Identify “black holes” in your market coverage. What accounts are you missing out on? Make sure you're engaging every account in the A group, and look for accounts your team could be working in the B and C segments.
3. Inject flexibility in account assignment. To ensure adequate coverage, you will likely need to retrieve accounts reps can't or shouldn't work right now, and give them more and better accounts to work.
Identifying and scoring an account's fit
Deciding whether or not a prospect is a good fit for your solution sounds like a simple, straightforward task. But even a simple ICP fit score can be far more complex than it initially appears. Let's start by understanding your ICP.
Ideal customer profile
An ideal customer profile (ICP) describes the attributes of the customers who are likely to need your product or services and would therefore be a very good fit for becoming a customer. For B2B companies, your ICP will likely the size and key firmographics of prospect accounts that would have a need for your product.
Use your ICP to properly target marketing and sales activities to the right kinds of prospects.
Ensure your ICP is well-defined and precise
Start by conducting a thorough analysis of your existing customer base, identifying the common traits, characteristics, and attributes of your most successful and valuable customers. Leverage data-driven insights and feedback from your sales team to refine and validate your findings. It's essential to go beyond basic demographics and delve into firmographics, such as company size, industry, and location, as well as behavioral indicators, such as buying patterns, pain points, and specific needs your product or service addresses.
Continuously review and iterate on your ICP as your business evolves, and leverage technology and analytics to gather real-time data and insights. By ensuring your ICP is well-defined and precise, you'll empower your sales team to target the right prospects, personalize their approach, and drive higher conversion rates and revenue growth.
Evaluate the firmographics in your ICP
Typically we think about B2B segmentation in terms of a few main criteria: revenue, location, industry and employee count. These broad categories help sales reps approach companies that are similar to each other in some basic way. The goal is to reduce complexity in the sales process.
But these criteria are broad strokes at best. Revenue and employee count are rough estimates of a company’s size, and thus its maturity. That tells us something about its challenges - a 20-person startup with $1 million in annual revenue has far different business challenges than a 4000-person company with $1 billion in revenue.
Location matters only a little to most sales teams, and generally in the more general sense. Is a prospect located in this country or region? That helps us know what language, time zone, and cultural implications we need to take into consideration. But as we’ve discussed many times, more fine-grained geographic criteria (such as state or zip code) is a terrible way to segment accounts. Opportunity is not distributed geographically.
With geographic segmentation, some reps get territories with so many high-potential accounts they can’t talk to them all, while other reps starve in a territory plan with low potential.
Beyond high-level regional considerations, geographic segmentation is not a particularly useful criterion. More on territory distribution coming up.
That leaves us with industry. Typically, we tend to cluster prospects into broad industry categories - software, automotive, healthcare, and the like. Those are moderately useful, but would be far more useful if they were more specific. Some companies use NAICS codes to segment account groups, but those codes are not much better than broad industry categories.
This is an area where newer AI technologies can help. We can use AI to take industry from a broad, high-level category to something more nuanced and operationalizable.
Imagine an AI analysis of what those accounts do, how they talk about themselves online, the way that they position the problems they solve, their products, and their customers.
We can take all that information and then create a bottoms-up map of account clusters based on similarities. So instead of trying to apply a top-down broad categorization, we're looking at the categorization that emerges from the data to show us how similar one account is to the next based on how they actually talk about what they do. This allows B2B companies to break their audience into much more useful and relevant segments based on industry. (If this sounds interesting to you, check out Market Map.)
Evaluating an account's timing
Even the perfect accounts may not be ready to buy. There are internal and external signals you can identify that tell you how likely an organization is to buy now.
Accounts are either ready and in market for a solution like yours (they don’t currently have a vendor for the problem you solve or their contract is almost up, they have budget, something’s changing in their company that you can help with) or they’re not (they just signed with another vendor, they’ve had to cut costs, organizational change isn’t pointed in your direction).
Here are some signals you can use to help determine an account's timing:
-
Inbound leads: If an account raises their hand asking for a demo, that’s a strong signal that they’re looking to buy soon.
-
ABM or intent data: If you use an ABM tool, you can get intent or buying stage data on prospect accounts to tell you when someone is likely to be ready to buy. More on intent shortly.
-
Point of contact (POC) changes: If a company hires a new person in a key leadership role, this may indicate they’re looking for a new solution.
-
Funding announcements: When a company raises new capital, they often tend to scale business processes, and this may signal they’re moving in-market.
-
Competitor renewal date: When you know when a competitor contract is up with a prospect, you can use this information to reach out a few months in advance.
-
“Get back to me” date: Sometimes prospects tell you they’re not ready for your solution now, but they ask you to get back to them in a few months. Make a note of that in your CRM and use it as a timing signal.
-
Marketing automation scores: If a prospect reaches a certain score threshold in your marketing automation platform (MAP, that may indicate they’ve moved in-market.
-
Scraping or proprietary data sources: Other tools and firms purport to give you additional information about prospects to help you find the right accounts at the right time.
A deeper dive into intent
An important part of prioritization is understanding an account’s intent, whether or not an account is currently in-market for your solution. A prospect that indicates some kind of intent to buy is far more valuable than a totally cold prospect. They have higher potential to convert.
We all love hand raisers - they’re the low-hanging fruit of intent signals. Those signals are when a prospect volunteers - or raises their hand - to learn more about your solution. That could be a demo request form fill, sales meeting scheduled, trial request, etc… Hand raisers are expressing high intent and should be prioritized accordingly.
However, there’s a whole universe of other ways prospects can indicate softer or quieter intent. For example, you can look at marketing activities, like visiting the pricing page 3 days in a row, watching a product demo video, engaging with a product tour. There are also intent data providers, like 6sense, that look at other signals to help you understand a prospect might be in-market right now.
Why intent signals matter
Prioritizing prospects based on intent to buy ensures your reps focus their energy on the most important accounts. They’ll spend less time on low-value prospects or manually sorting lists to target. It has a few other benefits as well.
Increasing your return on advertising spend. Using intent signals allows you to concentrate marketing spend on accounts based on their intent within the funnel. This shortens sales cycle time and keeps your prospect interested. You can also target different intent stages with different content and messaging.
Lowers your customer acquisition cost. Targeting prospects with the right information during their customer journey overwhelmingly lowers the cost of chasing leads that don’t fit your ICP and aren’t showing valuable signals. For example, a good retargeting campaign on video and/or content moves a prospect farther down the funnel than your awareness campaign push.
Identifies PLG leads. Intent signals aren’t only for new business either. You can use product usage signals to understand a customer’s potential for expansion. Are they adding new users? Using particular features that are connected to higher growth potential? Product-based signals can help you find customers that might be ready to buy more, which can help meet revenue goals.
Reduces churn. Is your customer viewing the cancellation page? Are they doing product research for competitors? Proactively reaching out to resolve issues and find product gaps creates a better customer experience and higher customer satisfaction.
One of the big mistakes we see companies make with using intent signals in a vacuum. Intent alone is not enough to determine if an account is likely to buy your product. But intent signals combined with fit data give you a much more reliable estimate. That's where account scoring comes in.
Account scoring
This leads us to account scoring. That’s the process of taking all these fit and timing signals and creating a score that indicates the priority level of an account. Reps should always be working the highest priority accounts available. Conversely, every high potential account should be worked.
Building an account scoring model
So, how do you develop an account scoring model?
Everything that follows assumes you're using an account-based sales motion, so we're talking about how to score accounts. But these concepts can apply to lead scoring as well.
You can score accounts on a numerical scale (like 1-10) or group them into tiers (like A, B, C or high, medium, and low priority). Or you may first score them with a specific number, then group those scores together into tiers. For example, anyone with a score of 8-10 goes into Tier 1, accounts with 5-7 go into Tier 2, and those with a score of 4 or below are Tier 3. Pick whatever scoring system makes sense for your company.
But the real question is - what methodology do you use to develop your account scores? Let's talk about some of the possible B2B account scoring with examples.
The types of account scoring models
The most common B2B account scoring models are:
1. Rule-based models
These models use a set of rules to score accounts. The rules are based on factors such as company size, industry, and location, and have been manually created by humans.
Rule-based models are the simplest to create and what most companies start with, but are likely not as accurate as statistical or machine-learning models. The rules for these models could be based on a number of factors, including
- Firmographic rules: These rules are based on an account’s firmographic or technographic characteristics, such as company size, industry, location, or technology use. These are ICP fit rules.
- Behavioral rules: These rules are based on an account’s behavior, such as website visits, marketing activities, social media engagement, and email engagement. These rules tend to attempt to identify high-intent behaviors to surface accounts that are currently in-market for a solution. These are ICP timing rules.
Firmographic rules are a good starting point for scoring accounts because they’re relatively simple to set up and automate with basic data enrichment. However, those rules can be less accurate than other kinds of rules, as they don’t take into consideration the individual behavior of each account. Behavioral or intent rules can be more accurate, especially when paired with firmographic rules, but intent data can be harder (and more expensive) to come by. Behavior rules may also require some kind of real-time automation beyond simple data enrichment.
We recommend starting simply with a few firmographic rules, paired with some behavior rules to fully capture an account’s fit and timing. This will give you the highest success rate at identifying the highest-potential accounts.
2. Statistical models
These models use statistical algorithms to score accounts. The algorithms are trained on data from past sales and marketing campaigns. These are more complex to set up and will require some specialized statistical experience and knowledge on your team.
3. Machine learning models
These models use machine learning algorithms to score accounts. The algorithms are trained on data from past sales pipeline data, marketing campaigns, as well as data from other sources, such as social media and customer reviews, to create an account score based on previous accounts’ lifecycles. This will likely require a separate machine-learning product or an ML developer to implement. Because of this, it's a more advanced model and better suited for more mature companies.
4. Hybrid models
These models combine two or more of the above models. For example, a hybrid model might use a rule-based firmographic model to score accounts initially, and then use a machine learning model to refine the scores over time.
Choosing the right scoring model
Here are some of the factors to consider when choosing a model:
-
Data availability: Select a model that can use the data you currently have available to your business.
-
Accuracy: The model should be as accurate as possible in predicting which accounts are most likely to buy. Take the time for historical data analysis to fully understand what criteria are highly correlated to a successful customer.
-
Complexity: The model should be easy to understand and implement. The more complex the model is, the harder it will be to set up and maintain.
-
Cost: The model should be affordable. If you can’t afford an expensive intent data provider, start with a simpler scoring model that uses data you already have.
A rules-based account scoring model example
At Gradient Works, we've evolved our own account scoring method over time. We're still relatively early stage, so our model is a simple rules-based model for now. As we grow, we'll incorporate machine learning into the model, but we're not there yet. Still plenty of room to improve our rules manually first.
We started with a set of firmographic rules to create our initial account score. You can even use a simple spreadsheet to calculate these scores, then input them into a field on the account object in your CRM (if you're account based, that is - you may prefer to put it on the lead or contact object, depending on how you assign prospect accounts to sales reps).
We sell to high-velocity B2B sales teams, so a few of our initial account scoring criteria were:
- Is a B2B company
- Has 25 or more sales reps
- Sells a subscription product
- Has a self-service or free trial option
- Has an ASP of $1,000 - $100,000
A company got 1 point for each of these criteria they matched, and the higher the score, the higher potential we considered that account. These simple criteria helped us identify accounts that would have a complex and large enough sales cycle to need our dynamic books software. They're also very simple to identify by quickly looking at a company's website, Zoominfo profile or LinkedIn profile.
As we matured the model, we refined the firmographic and technographic criteria we look for. A company has to use Salesforce as its CRM to use Gradient Works right now, so that doesn't go into our scoring criteria (it's a binary qualified/unqualified signal), but we do look for companies that use other sales tech, like an intent provider such as 6sense. A company doesn't have to use 6sense to use Gradient Works, but many of our customers do, so it's a positive indicator of success. Accounts get a point for that.
We also added behavior/intent rules to our scoring model. For example, accounts that have visited our website multiple times over multiple sessions, have multiple contacts engaging with our marketing materials, or visit high-intent pages on our website (such as demo request and product tour pages) get points for those behaviors.
We then assign new high-score accounts to sales reps automatically using our very own product (Gradient Works), so that our sales team always has the most current and highest-potential accounts in their books at all times. It helps us call on accounts that are both high fit and actively signaling their intent.
That's how we started, and it's a great place for anyone to start. Start simple and small, and iterate your way to something more complex as you learn how accurate your scoring is.
Keep these guidelines in mind as you go.
1. Start simple
If you're just getting started with account scoring, then we recommend a super simple rules-based model to start. Put it in place for a few months, then go in to review and see what you should tweak.
You probably have some sense of what firmographic criteria matter more than others, for example. You probably also know what intent signals are more important. Weight those higher. Just keep the math super simple. Know that the data you have won't always be perfect. And even if your weights are right, you may be weighting the wrong values. But you need to start somewhere, so just start. You can - and should - improve your model as you go.
Account scoring can be as straightforward or as complicated as needed to help prioritize your lists. A simple cumulative score is effective and easy to build quickly. As companies scale, they often begin weighting both firmographics and lead activity to build a score. Two examples:
Example 1: Firmographics/technographics score
- Simple: +1 point if the account uses marketing automation software
- Weighted: +3 points if they use Marketo because you have a direct integration vs. +1 for Eloqua because there is no integration yet
Example 2: Lead intent signal
- Simple: +1 when an account visits a high-intent page (demo, pricing)
- Weighted: +3 points for demo visit, +2 for pricing
As your company or sales team matures, then you can integrate more complicated models like a statistical or machine learning model. Ideally, you'd do these in conjunction with your rules-based model to create something accurate (and always improving in accuracy), but also relatively easy to understand why an account scores the way it does.
2. Aim for progress over perfection
The first version of your account score may not be perfect, but it won’t be useless. It'll be directional and it'll help reps prioritize. That's a win! Next, make it better.
Build improvement into the process. Enable reps to report issues and swap out an account if there's a problem (more on returning accounts in the next section). When you get this feedback, act on it. Review and respond to the rep. Feedback without action means no more feedback.
There are a lot of assumptions made here based on data. Data changes. The score is not set in stone. Review it at least once a quarter, and make adjustments as necessary. Have the highly-scored accounts turned into qualified opportunities? You'll likely find some things you should include that you didn't at first, as well as some things you can remove from your score entirely.
Quickly identify and automatically distribute high potential accounts to reps with Gradient Works
Improve efficiency on your sales team with an automated, dynamic approach to account prioritization and distribution.